AI has emerged as a fundamental technology for the banking and financial services industries, with best-in-class organizations recognizing its vital importance to their success. Over 300 executives, analysts, and data scientists packed the ballroom at the Conrad New York on Wednesday, June 20 to learn firsthand just how to become an AI-driven enterprise, and why that evolution is so critical, particularly in these industries.
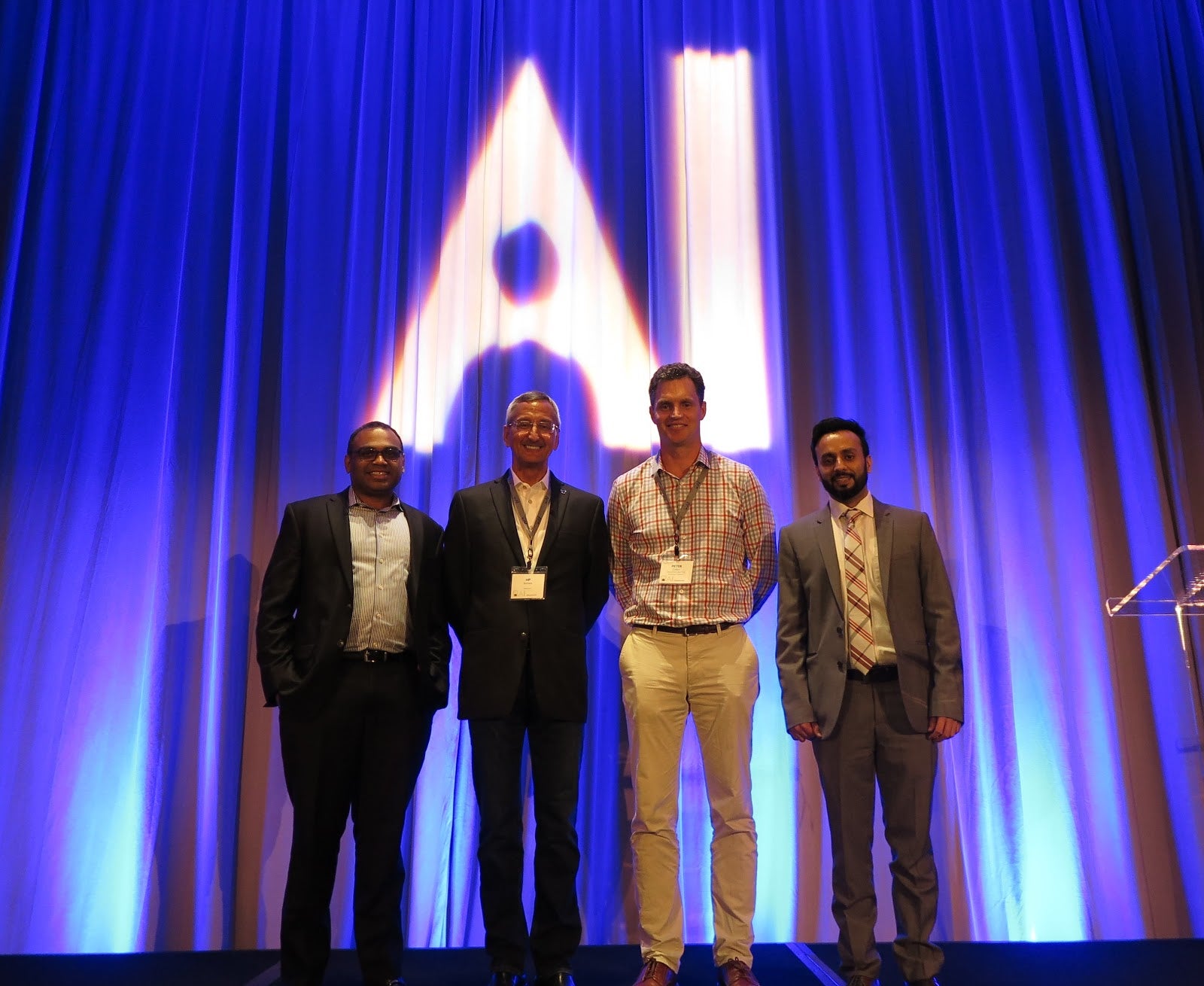
Paras Parekh (Credit Suisse), HP Bunaes (DataRobot), Peter Cotton (JP Morgan), Anshul Sehgal (Credit Suisse)
After an opening keynote from DataRobot’s GM of Banking Greg Michaelson, J.P. Morgan’s Executive Director Peter Cotton explored the rise of data science, and what that means at J.P. Morgan Chase specifically. Working with DataRobot has made building and evaluating models much easier, opening up a world of “loud” problems to solve across various banking sectors.
Peter was followed by DataRobot’s Director of Banking, H.P. Bunaes, who echoed Peter’s thoughts and emphasized the numerous banking use cases that could be efficiently and effectively solved using automated machine learning.
Paras Parekh, the Director – Head of Global Markets Predictive Analytics at Credit Suisse, and his colleague Anshul Sehgal, a Senior Data Scientist, wrapped up the proceedings with an in-depth breakdown of how machine learning is giving Credit Suisse an edge through differentiated research.
With over 2.5 quintillion bytes of data being created every day, active fund managers at Credit Suisse recognize the potential within all of this newly created data.
They also dove deep into one specific use case, before emphasizing that data scientists are not made redundant by automated machine — on the contrary, their focus shifts slightly, but their value remains higher than ever before.
AI Experience Speaker Spotlight: Paras Parekh and Anshul Sehgal from Credit Suisse
Paras and Anshul kicked off their session by leading the audience through a quick lesson about the differences between supervised learning, unsupervised learning, and reinforcement learning, before testing the audience with an interactive pop quiz. After getting the audience warmed up, they dove into the meat of their presentation: “Creating Differentiated Research through Machine Learning.”
With over 2.5 quintillion bytes of data being created every day, active fund managers at Credit Suisse recognize the potential within all of this newly created data. These new and alternative datasets — unique information about a company (that represents a potential investment opportunity) published by sources outside of the company — have tremendous potential to generate alpha, with hedge fund spending on alternative datasets projected to grow to $7 billion by 2020. For Credit Suisse, this is the type of data and analytics that should be powering financial research today, and in the future.
Paras and Anshul dove deep into one specific use case they’ve tackled by using DataRobot’s automated machine platform: How to improve Credit Suisse’s valuation of an oil exploration and production company, as an investment opportunity. For this valuation, Paras and Anshul were trying to predict this company’s future oil production.
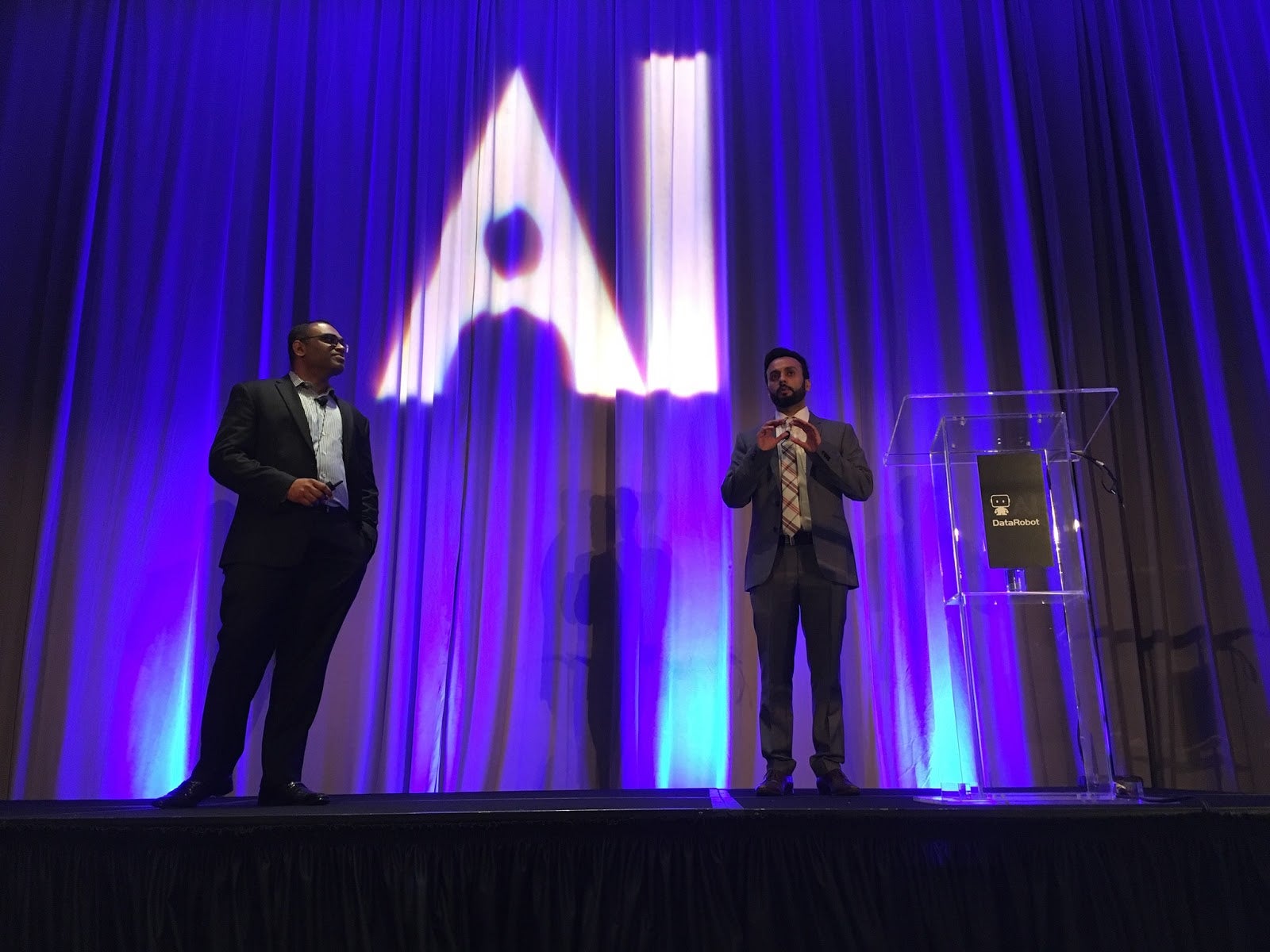
Paras Parekh and Anshul Sehgal (Credit Suisse)
They started with the data, collating and blending both traditional and alternative datasets. Some of the publicly available alternative data they included in their datasets included well locations and well production data from DrillingInfo.com, propane and water usage data from FracFocus.com, and well permit data from the US Government.
In walking the audience through the model building process, Paras and Anshul spotlighted the power of DataRobot in expediting the overall timeline of the project. After collecting their datasets, the steps for data preparation remained the same even after using DataRobot: data scientists working with analysts to cleanse the data, remove noise, and figure out what to do with missing values and outliers.
Paras recommended integrating data preparation and extraction tools as part of the data ingestion and processing step in your functional architecture, using tools like Cloudera and Alteryx. Once the data had been cleaned, the data scientists and business analysts worked through exploratory analysis to identify and generate features. Overall, these initial steps of the model building process take 3-4 weeks, both with and without DataRobot.
However, when going through the next step, the power and potential of DataRobot was made abundantly clear to the audience. After cleansing and prepping the data with analysts, historically the data scientists at Credit Suisse would then go away to build and test the models on their own. This process used to take at least 2 weeks: by using DataRobot, that process now takes only 2 hours.
DataRobot’s automated machine learning platform does not replace data scientists, and their ability to build and test models; Anshul and Paras stressed that what DataRobot does is enhance a data scientist’s abilities, acting as an elite decision support system and providing massive productivity gains.
Even with a tool like DataRobot automating the machine learning model building and testing process, the golden rule still applies to data scientists: garbage in, garbage out. Without a data scientist who truly understands the data and how to prep it, you’re going to put garbage into your automated machine learning models and get garbage predictions out. The process still fails without a data scientist.
Data scientists at Credit Suisse, working with DataRobot’s platform, have seen their roles slightly shift to focus on two critical parts of the model building process:
-
Model selection and analysis. After the informative features have been identified and generated, it’s imperative for data scientists to work on model selection, model analysis, and hyperparameter tuning within DataRobot. With this work on feature engineering, Paras and Anshul are able to develop highly accurate models that, when combined with the cleansed data and the engineered features, can deliver “perfect predictions.”
DataRobot’s automated machine learning platform does not replace data scientists, and their ability to build and test models; Anshul and Paras stressed that what DataRobot does is enhance a data scientist’s abilities, acting as an elite decision support system and providing massive productivity gains. Using DataRobot merely shifts a data scientist’s focus away from manual, time-consuming processes to focus on other more creative and value-add aspects of the model building and testing process.
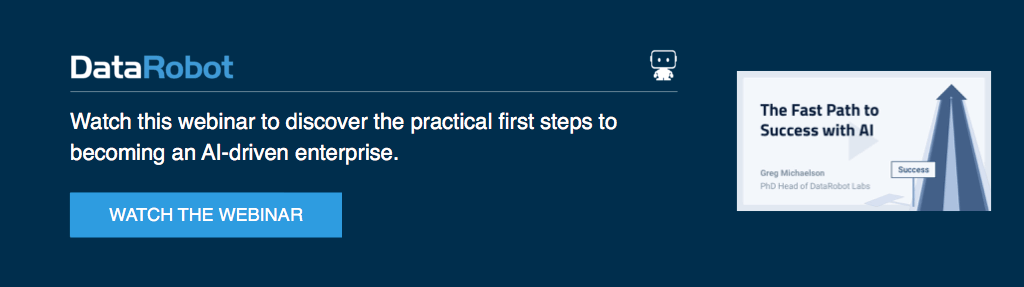
About the author
Gareth Goh is the Customer Marketing Manager at DataRobot. He was previously the Web Director at DataRobot, and has also worked at several different tech startups in the Boston area in various marketing roles. Gareth has an M.S. in International Relations and a B.S. in Journalism from Boston University.
Meet Gareth Goh