Colin Priest is a Fellow of the Institute of Actuaries of Australia and has worked in a wide range of actuarial and insurance roles, including Appointed Actuary, pricing, reserving, risk management, product design, underwriting, reinsurance, relationship management, and marketing. Over his career, Colin has held a number of CEO and general management roles, where he has championed data science initiatives in financial services, healthcare, security, oil and gas, government and marketing. He frequently speaks at various global actuarial conferences.
Colin is a firm believer in data-based decision making and applying machine learning to the insurance industry. He is passionate about the science of healthcare and does pro-bono work to support cancer research.
The great architect William Kent, once said “Nature abhors a straight line,” and this was reflected in his designs, particularly his landscapes and gardens. So if straight lines aren’t common in nature, then why do actuaries use straight line models?
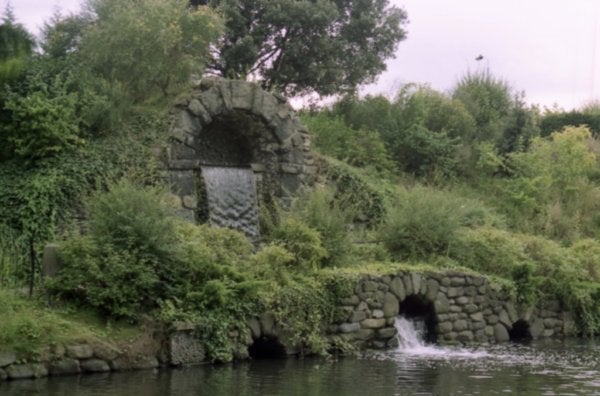
Photo: “Chiswick Gardens” designed by William Kent
Source: Patche99z – Own work, CC BY-SA 3.0
The generalized linear models (GLMs) that actuaries commonly use were first developed back in the 1970s, a time when computers were not powerful and data was small. It’s embarrassing to admit, but I’m old enough to remember those times when I had to use logarithm tables because pocket calculators were not commonly available! GLMs were designed for quick and simple computational cost because that was all that was available at the time.
I first used GLMs for insurance pricing in the 1990s. While computer power had grown exponentially over the two decades since GLMs were first developed, my computer still took hours to fit GLMs.
But even though my 2017 computer can fit a GLM in seconds, that doesn’t mean that building pricing models with GLMs is a quick process taking mere seconds of my time.
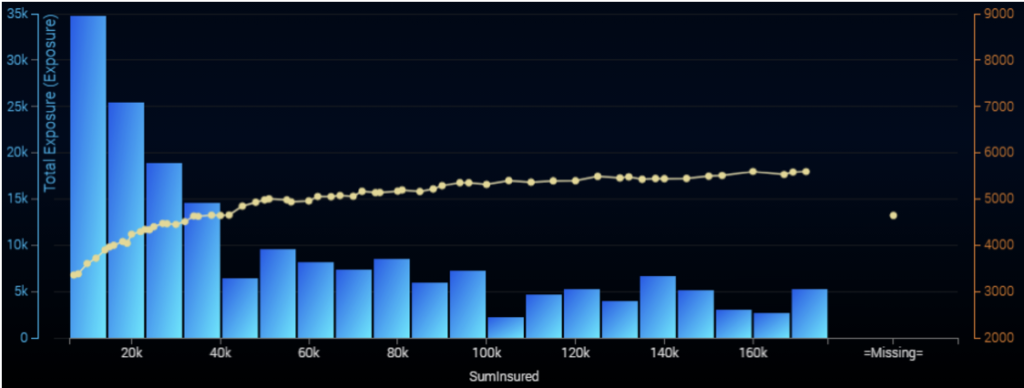
GLMs assume that the relationship between a rating factor and claim costs is a straight line. But look at the plot above showing a relationship (the yellow line) between auto/motor insurance claim costs and the sum insured on the insurance policy. That isn’t a straight line! If we fitted a GLM to this data, we would have built a weak model that mispriced the insurance policies.
Actuaries spend a lot of time looking for the right mathematical transformations for rating factors, to turn a crooked line or a curve into a straight line. This requires a lot of repetitive manual coding, experimentation, iterative improvements, and a lot of valuable time. Actuaries don’t have time to test all of the possible patterns and mathematical functions, so they stick with what they are comfortable with. This often results in models that could be improved, if only they had more resources available to them. Some insurers build huge teams of pricing analysts whose job is to manually search through mathematical functions to find incrementally better models.
Whenever I see a repetitive manual process like this, I see an opportunity for efficiency via automation. Just like we no longer manually copy books, we shouldn’t have to manually try to straighten real-life data into artificial straight lines.
Modern machine learning algorithms are designed for modern, powerful computers and bigger data. They don’t make restrictive assumptions that the world is full of straight lines. Instead, they adjust equations automatically, to find the best patterns, trying hundreds of thousands of shapes and mathematical functions. They test these patterns against independent validation data, not the data they were trained on, to ensure that the patterns remain valid for new data. This is a much more robust approach than the manual processes that actuaries have been using, and actuaries are starting to realize this and embrace new machine learning insurance technology.
DataRobot makes this easy for me. Here’s what DataRobot does automatically for me:
- Builds hundreds of different algorithms on your data
- Applies hundreds and thousands of patterns to your data
- Tests which algorithms and patterns work best on data that they have never seen before
- Scales back patterns that are not based upon credible data
When I tell DataRobot to do this mundane, time-consuming work for me, it does so with the mere click of a button. DataRobot finds the best patterns with more accuracy than I ever could, in much less time. Now I can focus my efforts on commercial considerations, growing sales and profit, instead of sitting in front of a computer playing around with numbers and equations.
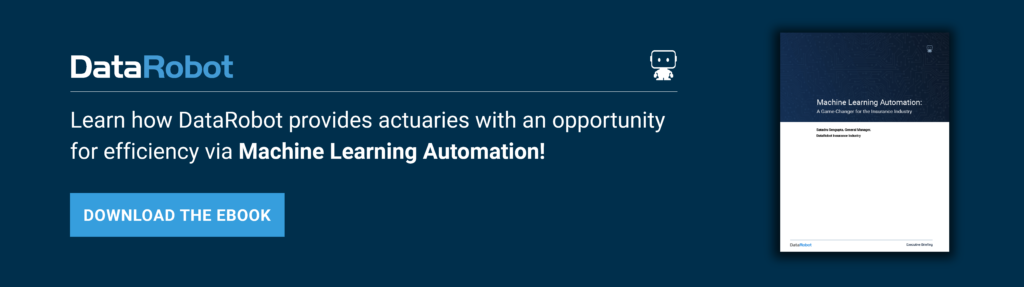
About the author
Colin Priest
VP, AI Strategy, DataRobot
Colin Priest is the VP of AI Strategy for DataRobot, where he advises businesses on how to build business cases and successfully manage data science projects. Colin has held a number of CEO and general management roles, where he has championed data science initiatives in financial services, healthcare, security, oil and gas, government and marketing. Colin is a firm believer in data-based decision making and applying automation to improve customer experience. He is passionate about the science of healthcare and does pro-bono work to support cancer research.
Meet Colin Priest